
Understanding and Overcoming Artificial Intelligence Bias
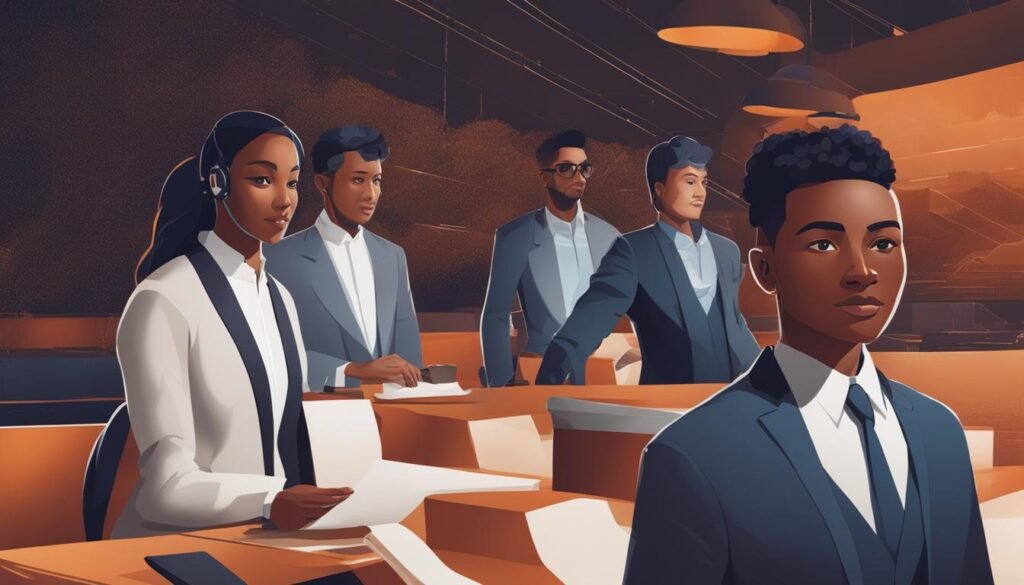
Greetings, readers! Today, I want to delve into the intriguing world of artificial intelligence (AI) and explore a topic that has been gaining immense attention recently - AI bias. As we rely more on AI algorithms to make critical decisions in various domains, it is crucial to understand and address the biases that can creep into these systems.
AI bias refers to the inherent prejudices that can be embedded in the algorithms, leading to biased outcomes and perpetuating unfairness in AI. Biased algorithms can have far-reaching consequences, from reinforcing societal stereotypes to impacting real-world scenarios like wrongful arrests.
In order to ensure fairness and algorithmic fairness in AI systems, it is essential that we work towards mitigating bias and promoting ethical AI. Detecting and eliminating bias in AI is a complex task, but with dedicated efforts, we can overcome this challenge and create unbiased AI systems that benefit everyone.
See Also...
Key Takeaways:
- AI bias can perpetuate stereotypes and discrimination.
- Biased AI systems can have serious real-world consequences.
- Fairness and algorithmic fairness are essential in addressing bias in AI.
- Implementing bias detection mechanisms can help mitigate biases in AI.
- Collaboration between developers, researchers, and policymakers is crucial in overcoming AI bias.
Stay tuned for the next section, where we will explore the issue of bias in artificial intelligence in greater detail.
Remember, AI has immense potential, but it is up to us to ensure it serves us all fairly!
- How can AI and edge computing revolutionize healthcare?
- What is the role of early health prediction in improving public health?
- How can edge AI enhance early health prediction?
- What are the consequences of biased AI systems?
- How do AI systems learn biases?
- Are current solutions effective in addressing algorithmic bias in AI?
- How can bias in AI be overcome?
- What is the ongoing research focused on?
The Issue of Bias in Artificial Intelligence
Artificial intelligence (AI) has become increasingly integrated into our daily lives, from personalized recommendations on streaming platforms to voice assistants that help us navigate our day-to-day tasks. However, one pressing concern that arises with AI is the issue of bias embedded within these systems. Biased algorithms can perpetuate stereotypes and discriminations, leading to real-world consequences such as wrongful arrests and the perpetuation of societal biases.
See Also...
AI systems learn from human-generated data, which means that any biases present in the data can be learned and reinforced by the algorithms. This creates a self-perpetuating cycle of bias in AI that can be challenging to overcome. While there have been efforts to address algorithmic bias in AI through the use of fair and diverse databases, these solutions have limitations and may not fully solve the problem.
As AI continues to play a crucial role in various domains, it is imperative that we find comprehensive approaches to address and mitigate bias in AI and machine learning. This requires a collaborative effort between AI developers, researchers, and policymakers. Ongoing research is focused on developing strategies that reduce bias in AI and improve algorithmic fairness. Additionally, implementing bias detection mechanisms in AI systems can help identify and mitigate biases, ensuring greater fairness and ethical considerations in AI.
Key Takeaways:
- Biased AI systems can perpetuate stereotypes and discriminations, leading to real-world consequences.
- AI systems learn biases from human-generated data, creating a self-perpetuating cycle of bias in AI.
- Current solutions to address algorithmic bias have limitations and may not fully solve the problem.
- Comprehensive approaches and collaboration are needed to effectively address and mitigate bias in AI and machine learning.
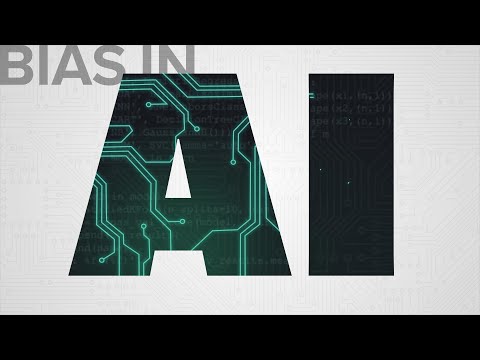
Types of Bias in AI | Impact |
---|---|
Gender bias | Reinforces gender stereotypes and discrimination. |
Racial bias | Contributes to racial profiling and unequal treatment. |
Socioeconomic bias | Perpetuates socioeconomic disparities. |
Age bias | Leads to age discrimination and exclusion. |
Strategies for Overcoming Artificial Intelligence Bias
When it comes to artificial intelligence (AI), fairness and algorithmic fairness are essential to ensure unbiased outcomes. As we continue to unlock the full potential of AI, it becomes crucial to address and reduce bias in AI systems. To achieve this, ethical AI principles and guidelines play a significant role in mitigating bias and promoting fairness. By adhering to these principles, we can create AI systems that are accountable and equitable.
See Also...
One effective strategy for reducing bias in AI is the implementation of bias detection mechanisms. These mechanisms help identify and flag instances of bias in AI systems, allowing developers to actively mitigate and rectify them. Ongoing research is dedicated to improving these detection methods to ensure better algorithmic fairness in AI. By continuously enhancing our ability to detect and address bias, we can create AI systems that are more reliable and trustworthy.
However, overcoming bias in AI requires collaboration. AI developers, researchers, and policymakers must work together to address the challenges and intricacies associated with bias in machine learning. By collaborating, we can develop holistic approaches that tackle bias at its core, ensuring that AI systems are fair and unbiased. This collaborative effort will foster innovation and drive progress towards a more equitable AI landscape.
In conclusion, fairness in AI, algorithmic fairness, ethical AI, and bias detection are key strategies for addressing and overcoming bias in artificial intelligence. By prioritizing these aspects, we can build AI systems that are not only powerful and efficient but also fair and just. Together, we can shape an AI-driven future that benefits everyone and upholds the principles of fairness and equality.
FAQ
How can AI and edge computing revolutionize healthcare?
AI and edge computing have the potential to revolutionize healthcare by enabling disease detection and prediction, analyzing healthcare data, and developing targeted interventions. This can improve public health outcomes, lower healthcare costs, and enhance the patient experience.
What is the role of early health prediction in improving public health?
Early health prediction plays a critical role in improving public health by identifying and addressing potential health problems before they worsen. It can help prevent chronic diseases and reduce healthcare costs.
How can edge AI enhance early health prediction?
Edge AI, which combines AI and edge computing technologies, has the potential to enhance early health prediction by bringing computing power close to the data source. This enables real-time monitoring and decision-making in healthcare.
What are the consequences of biased AI systems?
Biased AI systems can perpetuate stereotypes and discriminations, leading to real-world consequences such as wrongful arrests and the perpetuation of societal stereotypes.
How do AI systems learn biases?
AI systems learn biases from human-generated data, creating a self-perpetuating cycle of bias in AI.
Are current solutions effective in addressing algorithmic bias in AI?
Current solutions to address algorithmic bias, such as fair and diverse databases, have limitations and may not fully solve the problem. Comprehensive approaches are needed to effectively address and mitigate bias in AI and machine learning.
How can bias in AI be overcome?
To overcome bias in AI, it is essential to ensure fairness and algorithmic fairness in AI systems. Implementing bias detection mechanisms and following ethical AI principles and guidelines can also help in addressing and reducing bias in AI.
What is the ongoing research focused on?
Ongoing research is focused on developing strategies to reduce bias in AI and improve algorithmic fairness. Collaboration between AI developers, researchers, and policymakers is crucial in addressing bias in machine learning and artificial intelligence.
Source Links
- https://www.mdpi.com/1999-5903/15/11/370
- https://gobookmart.com/many-a-book-is-like-a-key-to-unknown-chambers-within-the-castle-of-ones-own-self/
- https://webappia.com/the-prejudices-that-artificial-intelligences-are-inherently-programmed-with-aiprejudice/
If you want to know other articles similar to Understanding and Overcoming Artificial Intelligence Bias you can visit the Blog category.
Leave a Reply
Related Post...