
Understanding the Complex World of AI Knowledge Representation
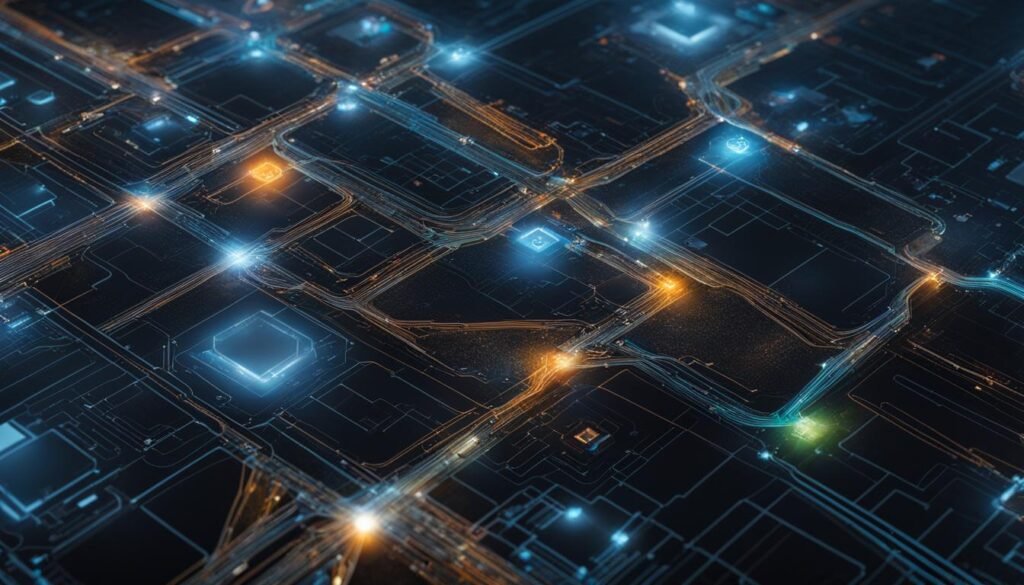
As the world increasingly relies on artificial intelligence (AI) to solve complex problems, various knowledge representation issues in artificial intelligence have come to light. These issues are particularly significant in fields like law, where AI legal dilemmas and concerns surrounding ethical AI usage play a critical role. As AI is used more in the legal field, including in court cases, lawyers and other legal professionals need to understand and adapt to these changes. They need to deal with issues like AI competence, AI implications, and AI technology adoption.
Key Takeaways
- Maintaining technological competence with AI developments in law is essential for legal practitioners.
- Understanding the benefits and risks of AI is crucial for responsible and ethical usage.
- A thorough grasp of AI's knowledge representation techniques can empower professionals to optimize its potential in various sectors.
- Some key elements of AI knowledge representation include knowledge bases, graphs, semantic networks, and ontologies.
- It is important for the success of AI applications to be able to deal with the problems that come up when representing knowledge in AI, like how to handle ethical issues when using natural language processing techniques.
- Collaboration between legal professionals and AI developers can lead to more responsible and effective AI tools in the legal industry.
- Continuing education about AI and knowledge representation can help professionals adapt to an increasingly AI-augmented landscape.
- What are the main challenges with knowledge representation in AI?
- How are NLP techniques relevant to knowledge representation in AI?
- What are the ethical considerations when using AI in the legal profession?
- How can legal practitioners adapt to the evolving AI landscape?
- What are the components of knowledge representation in AI?
- How do ontologies and semantic networks contribute to AI knowledge representation?
The Fundamentals of Knowledge Representation in AI
The core aspects of knowledge representation in AI encompass the creation and utilization of knowledge bases, graphs, semantic networks, and ontologies. These components form the structural backbone for various AI applications, especially in the field of natural language processing (NLP). The ability of AI to effectively represent, organize, and interpret complex data is fundamental to its success across varying contexts. This is clear because AI is being used more and more for complicated tasks like legal analysis. AI systems like CoCournsel show how specialized AI can help lawyers by giving them new and more accurate information.
See Also...
To gain a better understanding of the fundamentals of knowledge representation in AI, let us delve deeper into its primary components:
- AI knowledge bases in NLP
- Knowledge graphs in AI
- Semantic networks in AI
- Ontologies in artificial intelligence
“Knowledge representation in AI enables machines to understand, interpret, and reason in a way similar to humans.”
These components play a crucial role in different AI applications. To illustrate, consider the table below for an overview of their respective functions.
See Also...
Component | Key Features | Applications |
---|---|---|
AI knowledge bases in NLP | Store linguistic data and rules to aid in understanding, processing, and generating human language | Text analysis, sentiment analysis, chatbots, and machine translation |
Knowledge graphs in AI | Create informative visualizations of interconnected entities and relationships within a specific domain | Data integration, information retrieval, and recommendation systems |
Semantic networks in AI | Represent knowledge in the form of interconnected nodes and connective links that simulate human cognitive processes | Expert systems, knowledge organization, and automated reasoning |
Ontologies in artificial intelligence | Systematic representation of domain-specific knowledge, providing semantic clarity and logical structure to AI systems | Information retrieval, natural language understanding, and data integration |
A lot of different complex parts work together to make AI knowledge representation possible. These include knowledge graphs, ontologies, AI knowledge bases for natural language processing (NLP), and more. These have enabled AI to achieve remarkable success in processing varied and intricate data sets, impacting diverse fields such as legal analysis and beyond. Understanding these fundamentals helps bring us a step closer to realizing the full potential that artificial intelligence holds for transforming our professional and personal lives.

As AI keeps getting better, it gets harder to represent knowledge in a way that is both correct and moral. This is especially true in the areas of natural language processing (NLP), ontologies, and semantic networks. In this section, we will explore these challenges and discuss the implications they have for AI developers and practitioners alike.
Grasping the Intricacies of NLP Techniques
Advancements in NLP techniques for knowledge representation have significantly impacted the potential of AI in interpreting and generating human language. However, there are still problems with making sure that NLP outputs are accurate and reliable. This means that they need to be closely watched and able to adapt to changing language situations. An essential aspect of addressing these challenges is understanding the ethical use of NLP in AI. Practitioners must remain informed about the capabilities and limitations of such AI systems to prevent reliance on incorrect, hallucinated, or fabricated data.
See Also...
"ChatGPT lawyer" incident - A recent example where an AI-generated legal advice contained fabrications of law case references, raising concerns about the ethical use of NLP techniques in AI.
Tackling Issues with Ontologies and Semantic Networks
Ontologies in artificial intelligence and semantic networks play a crucial role in structuring AI knowledge. One of the primary challenges in AI knowledge representation is the refinement of these frameworks, which encapsulate complex relationships and terminologies. It is important to be very accurate and precise when building these frameworks so that AI systems can properly store and retrieve knowledge.
Knowledge Acquisition and Engineering: A Core Conundrum
When it comes to ethical and supervisory duties, knowledge acquisition and knowledge engineering are two of the biggest problems in artificial intelligence. Legal professionals and AI developers must collaborate to responsibly build AI tools and ensure users possess a clear understanding of the AI's operation. This collaboration is analogous to the supervisory role lawyers play with paralegals, where they are tasked with applying due diligence in verifying AI-generated output to prevent misinformation and uphold the integrity of the legal services provided.
See Also...
- NLP techniques for knowledge representation: ensuring accuracy and dependability of AI-generated language outputs
- Ontologies and semantic networks in AI: fine-tuning frameworks to encapsulate complex relationships and terminologies accurately
- Knowledge acquisition and engineering in AI: responsibility and supervision in AI development and application to maintain ethical standards and prevent misinformation
In conclusion, navigating the challenges of AI knowledge representation demands a deeper understanding of NLP techniques, ontologies, semantic networks, and the ethical responsibilities that come with it. By staying informed about AI developments and maintaining vigilance in supervising AI tools, we can steer clear of ethical pitfalls and ensure the effective implementation of AI technology across various industries.
Conclusion
When we think about how far artificial intelligence has come, it is clear that problems with representing knowledge, like how hard it is to use relational databases and ontologies, hold both great potential and possible problems. Legal practitioners and AI developers alike should strive to wield AI's capabilities ethically and competently, fostering a future where AI technology adaptation revitalizes the legal field as well as numerous other industries.
Professional responsibility with AI is of paramount importance in navigating this ever-evolving technological landscape. The onus falls on both legal professionals and AI developers to collaborate and uphold stringent ethical standards as they create and utilize advanced AI tools for insightful analysis and decision-making purposes.
See Also...
In sum, the exciting world of AI demands constant learning, vigilant oversight, and a steadfast commitment to ethical conduct from all its stakeholders. By adhering to these principles, we can collectively overcome the difficulties in knowledge representation and open the door to an artificial intelligence-driven future that is more effective, fair, and innovative.
FAQ
What are the main challenges with knowledge representation in AI?
The main challenges with knowledge representation in AI include refining ontologies and semantic networks, ensuring the accuracy and dependability of NLP outputs, and addressing the ethical and supervisory obligations involved in knowledge acquisition and engineering.
How are NLP techniques relevant to knowledge representation in AI?
NLP techniques play a crucial role in advancing AI's potential in interpreting and generating human language. Proper implementation of these techniques helps improve the AI's ability to effectively represent, organize, and interpret complex data across various contexts.
See Also...
What are the ethical considerations when using AI in the legal profession?
Ethical considerations in the legal profession involve transparency regarding the AI's application in client services, maintaining technological competence about AI developments, and exercising due diligence in verifying AI-generated output to prevent misinformation and uphold the integrity of the legal services provided.
How can legal practitioners adapt to the evolving AI landscape?
Legal practitioners can adapt to the evolving AI landscape by staying informed about AI capabilities and limitations, responsibly using AI tools, and engaging in ongoing education concerning relevant technologies, as emphasized by professional organizations like the ABA.
What are the components of knowledge representation in AI?
Key components of knowledge representation in AI include the creation and utilization of knowledge bases, graphs, semantic networks, and ontologies. These components form the structural backbone for various AI applications, particularly in the field of natural language processing (NLP).
How do ontologies and semantic networks contribute to AI knowledge representation?
Ontologies and semantic networks contribute to AI knowledge representation by providing structured frameworks that encapsulate complex relationships and terminologies. Accurate and precise construction of these frameworks is crucial for proper knowledge representation and retrieval within AI systems.
Source Links
- https://podtail.com/podcast/strat/
- https://podtail.com/podcast/speaking-of-litigation-podcast/
- https://www.jdsupra.com/legalnews/applying-today-s-legal-ethics-to-today-3294580/
If you want to know other articles similar to Understanding the Complex World of AI Knowledge Representation you can visit the Blog category.
Leave a Reply
Related Post...