
Unlocking Knowledge in Learning in Artificial Intelligence Today
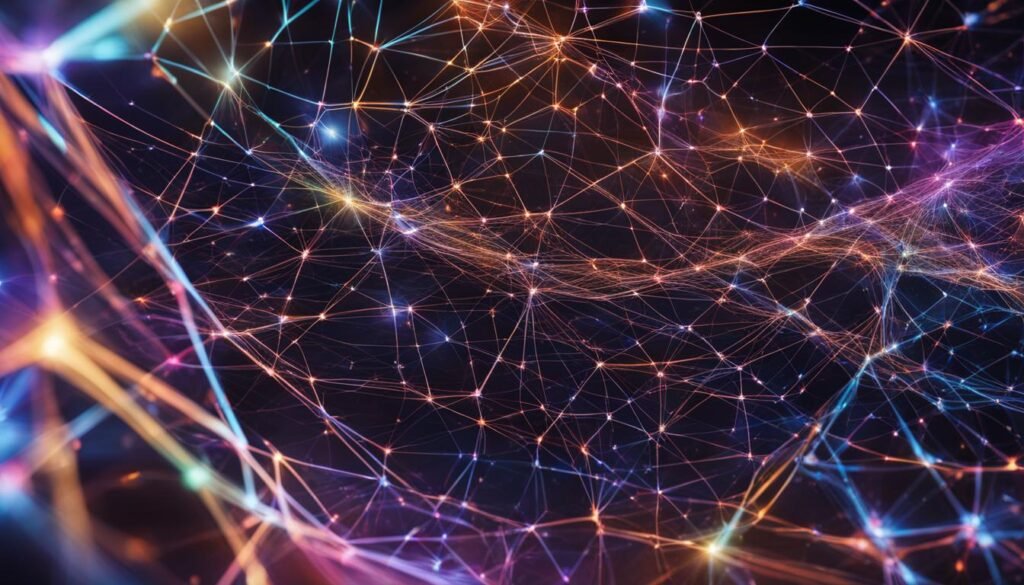
As the field of artificial intelligence continues to expand rapidly, the need for greater knowledge in learning artificial intelligence becomes ever more pressing. AI education is critical for building a solid foundation of understanding and fostering innovation within this rapidly evolving domain. In order to make artificial intelligence learning accessible to a wider audience, diverse educational opportunities and resources have been developed with the goal of demystifying this complex field.
Key Takeaways
- AI education is essential for nurturing a deeper understanding of artificial intelligence principles and methodologies.
- Interdisciplinary approaches to AI training can enhance one's understanding of AI and foster innovation by merging ideas from different fields.
- Real-world applications and case studies are valuable educational tools that demonstrate the practical use of AI and machine learning knowledge.
- Breaking down complex AI algorithms into understandable segments promotes a more inclusive learning environment.
- The ongoing quest for unlocking knowledge through learning in artificial intelligence is dynamic and ever-evolving.
The Landscape of AI Learning and Its Transformative Potential
AI education serves as a cornerstone for cultivating a deep understanding of AI principles and methodologies. Programs and courses aimed at explaining AI algorithms and theory are essential for building a skilled workforce capable of developing and implementing AI solutions. Structured knowledge paths are provided to ensure that learners can grasp fundamental concepts before advancing to more complex topics.
See Also...
Developing a strong foundation in machine learning knowledge and artificial intelligence learning is vital in today's rapidly evolving AI landscape. Foundational coursework empowers aspiring AI professionals with a well-rounded understanding of the essential principles that drive AI innovations.
Core Principles | Topics Covered |
---|---|
AI Algorithms | Supervised and unsupervised machine learning, neural networks, reinforcement learning |
Deep Learning Understanding | Convolutional neural networks, recurrent neural networks, generative adversarial networks |
Tools & Techniques | Python programming, TensorFlow, PyTorch, scikit-learn, Keras |
Interdisciplinary Approach to AI Training
To effectively capture the multifaceted nature of artificial intelligence, it is essential to integrate multiple disciplines into interdisciplinary AI training. This interdisciplinary strategy not only enhances one's understanding of AI but also fosters innovation by merging ideas from different fields. An interdisciplinary approach supports the development of versatile skills, encouraging students and professionals to approach AI challenges from a holistic perspective.
- Computer Science & Programming
- Mathematics & Statistics
- Data Analysis & Visualization
- Domain-specific Expertise (Health, Finance, etc.)
Case Studies: Learning AI Techniques Through Real-World Applications
Real-world applications act as valuable educational tools, illustrating the practical use of AI and machine learning knowledge. Through case studies, learners can study successful AI implementations, gaining insights into the practical challenges and solutions within the industry.
See Also...
IBM's Watson AI, for example, has been implemented in diverse applications, ranging from healthcare to customer service, showcasing the potential of artificial intelligence when applied across different sectors.
These case studies span various sectors and showcase the transformative potential of AI technologies when applied to real-world scenarios. By analyzing real-world AI applications, students can gain a deeper understanding of how AI techniques and algorithms can solve complex challenges and transform industries.
Breaking Down Complexities: Simplifying AI Algorithms for Broader Understanding

As the world delves deeper into the realm of artificial intelligence and machine learning, the need for simplifying AI algorithms becomes increasingly apparent. In order to cultivate a conducive learning environment for a diverse range of students and professionals, it's crucial to demystify the complexities of AI algorithms without compromising their essence. In essence, this involves breaking down complex algorithms into more digestible segments to facilitate understanding and pique curiosity.
See Also...
Fundamental to this process is the development of clear and concise explanations that enable learners to grasp AI and machine learning concepts more easily. Moreover, incorporating user-friendly tools into AI training programs plays an integral role in making these complex subjects more approachable.
"As we work towards developing a comprehensive understanding of machine learning and AI for all learners, the key lies in fostering an environment where curiosity thrives and simplicity reigns supreme."
To accomplish this, several key strategies can be employed, including:
- Using visual aids such as graphs, charts, and animations to illustrate abstract concepts.
- Creating engaging and interactive learning materials that encourage exploration and experimentation.
- Utilizing real-world examples and case studies to provide context and demonstrate practical applications of AI and machine learning.
One of the most effective ways to simplify AI algorithms is through the use of tables, which can convey complex information in a visually engaging and comprehensible format. For instance, consider the following table summarizing three popular machine learning algorithms and their respective features:
Algorithm | Type | Use Cases | Pros | Cons |
---|---|---|---|---|
Linear Regression | Supervised | Predicting numerical outcomes | Simple, widely applicable | Not suitable for non-linear relationships |
Decision Trees | Supervised | Classification and regression tasks | Interpretable, handles missing values | Prone to overfitting |
Clustering | Unsupervised | Discovering natural groupings in data | Finds hidden patterns, no need for labeled data | Requires careful choice of parameters and similarity measures |
In conclusion, the ultimate goal of simplifying AI algorithms is to foster a more inclusive and appealing learning environment, one that elevates the level of machine learning knowledge and empowers all learners—regardless of their background or prior experience—to become active contributors to the field of artificial intelligence.
Conclusion: Unlocking Knowledge in AI Learning
As we continue on our journey of unlocking knowledge in learning in artificial intelligence, it becomes clear that the landscape is dynamic and ever-evolving. AI education plays a vital role in providing a foundation that equips learners with the necessary skills and insights to contribute to and benefit from this rapidly growing field. Combining AI training, interdisciplinary education, and real-world applications, we strive to create a comprehensive learning environment for aspiring AI professionals.
To ensure optimal learning outcomes, it is essential to promote deep learning understanding among students and professionals alike. This is achieved by integrating multiple disciplines into AI training, encouraging learners to approach AI challenges from a broader perspective. Developing versatile skills allows individuals to drive innovation and solve complex problems within the artificial intelligence domain.
Finally, artificial intelligence learning must be adaptive and user-friendly. By simplifying AI algorithms and breaking down complex concepts into smaller, understandable segments, educators can foster a more inclusive and engaging environment. As the field of artificial intelligence advances, demystifying and disseminating AI knowledge becomes increasingly important, ensuring that people from all backgrounds and skill levels can harness the transformative potential of this powerful technology.
FAQ
What is the importance of AI education?
AI education is paramount as it develops the foundation for understanding and innovating within the field. Programs and courses aimed at explaining AI algorithms and theory are essential for building a skilled workforce capable of developing and implementing AI solutions.
What is the role of an interdisciplinary approach in AI training?
Integrating multiple disciplines into AI training enhances understanding and fosters innovation by merging ideas from different fields. An interdisciplinary approach supports the development of versatile skills, encouraging students and professionals to approach AI challenges from a holistic perspective.
How can we learn AI techniques through real-world applications?
Real-world applications act as valuable educational tools, illustrating the practical use of AI and machine learning knowledge. Through case studies, learners can study successful AI implementations, gaining insights into the practical challenges and solutions within the industry.
Why is simplifying AI algorithms important for broader understanding?
Demystifying the complexities of AI algorithms is crucial for broader comprehension and acceptance. Simplification does not mean diluting the science but rather providing clear explanations and user-friendly tools that make the concepts more approachable for learners of all levels.
Source Links
- https://techbullion.com/why-choose-tmgm-for-your-trading-journey/
- https://robots.net/tech/what-can-you-do-with-128gb-of-ram/
- https://webappia.com/econsultancy-introduces-new-short-course-on-ai-for-marketing-aimarketingcourse/
If you want to know other articles similar to Unlocking Knowledge in Learning in Artificial Intelligence Today you can visit the Blog category.
Leave a Reply
Related Post...