
Unlocking Potential with AI in Recommendation Systems
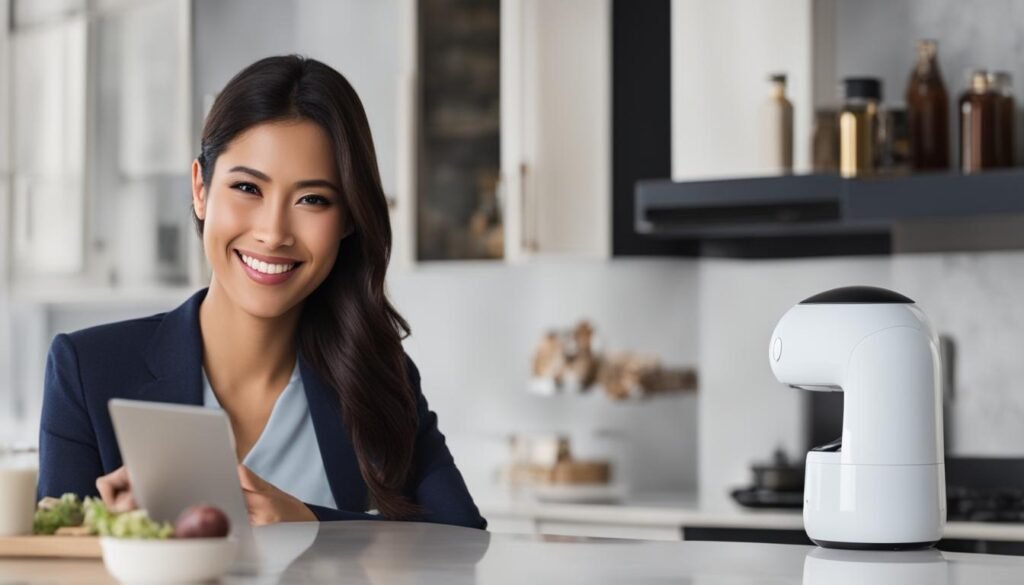
AI in recommendation systems is revolutionizing the digital experience by delivering personalized solutions. With the power of artificial intelligence, recommendation systems can analyze user behavior, preferences, and historical data to generate tailored recommendations. This technology utilizes machine learning algorithms, collaborative filtering, content-based filtering, and hybrid recommender systems to provide accurate and relevant suggestions to users. By leveraging AI in recommendation systems, businesses can enhance customer experiences, increase sales, and improve user engagement.
Key Takeaways:
- AI in recommendation systems delivers personalized solutions by analyzing user behavior, preferences, and historical data.
- Machine learning algorithms, collaborative filtering, content-based filtering, and hybrid recommender systems are used in recommendation systems to provide accurate suggestions.
- By leveraging AI in recommendation systems, businesses can enhance customer experiences, increase sales, and improve user engagement.
- Personalized recommendations are generated through the integration of AI and machine learning techniques.
- AI in recommendation systems has the potential to drive business growth and increase customer satisfaction.
The Role of NLP in Recommendation Systems
Natural language processing (NLP) plays a crucial role in recommendation systems. By leveraging NLP techniques, recommendation systems can interpret and understand user input, enabling the generation of personalized suggestions tailored to individual preferences and needs.
See Also...
NLP algorithms analyze the semantics and context of user data, extracting relevant information and matching it with appropriate recommendations. This process allows recommendation systems to provide accurate and contextually relevant suggestions, enhancing the overall user experience and increasing the likelihood of conversion.
For example, imagine a user searching for a specific product by describing it using natural language. With NLP, the recommendation system can extract key features and attributes from the user's query and match them with relevant products, delivering personalized suggestions that align with the user's preferences.
"NLP allows recommendation systems to understand and interpret user input, generating personalized suggestions that enhance the overall user experience."
In addition to search queries, NLP can also be used to analyze other forms of user-generated content, such as product reviews or descriptions. By extracting insights from this content, recommendation systems can further refine their suggestions and improve the relevancy of their recommendations.
See Also...
Benefits of NLP in Recommendation Systems
The integration of NLP in recommendation systems offers several key benefits. Firstly, it enables a more intuitive and user-friendly experience, allowing users to interact with the system using natural language instead of relying on structured search queries or filters.
Secondly, NLP allows recommendation systems to understand the intent behind user input, enabling them to generate personalized suggestions that align with individual preferences. This level of personalization increases the chances of user engagement and conversion, ultimately driving business growth.
Overall, NLP plays a vital role in enhancing recommendation systems by improving the accuracy, relevancy, and personalization of suggestions. By leveraging NLP techniques, businesses can unlock the potential to deliver personalized experiences and increase customer satisfaction.
See Also...
Deep Learning Advancements in Recommendation Systems
Deep learning has transformed the field of recommendation systems, providing powerful tools to deliver highly personalized recommendations to users. With the use of neural networks, deep learning algorithms can capture intricate patterns and relationships in vast amounts of data, enabling accurate and relevant suggestions.
One prominent application of deep learning in recommendation systems is the use of Convolutional Neural Networks (CNN). These networks excel at processing visual data, such as images, and can extract meaningful features that can be used to recommend relevant products or content. By analyzing images and understanding visual preferences, CNN-powered recommendation systems can present users with personalized suggestions that align with their tastes and preferences.
See Also...
Another key advancement is the utilization of Recurrent Neural Networks (RNN) in recommendation systems. RNNs specialize in processing sequential data, such as user behavior or text, and can identify patterns and capture temporal dependencies. By understanding the sequence of user actions or preferences, RNN-powered recommendation systems can generate personalized recommendations based on a user's historical interactions and preferences.
Benefits of Deep Learning in Recommendation Systems
The integration of deep learning in recommendation systems brings several benefits. Firstly, it enables the generation of highly personalized recommendations that cater to individual user preferences. By analyzing complex patterns and relationships in data, deep learning algorithms can provide accurate and relevant suggestions that resonate with users.
Secondly, deep learning allows recommendation systems to process diverse types of data, including text, images, and user behavior. This versatility enhances the system's ability to capture a comprehensive understanding of user preferences and generate recommendations across various domains.
Lastly, deep learning models can continuously improve and adapt based on user feedback. With techniques such as reinforcement learning, recommendation systems can optimize their performance over time, fine-tuning the recommendations to better meet the evolving needs and preferences of users.
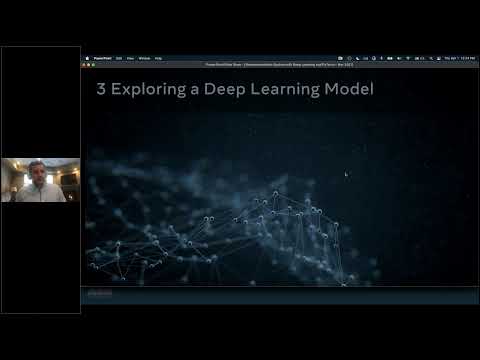
Table: Comparing Deep Learning Approaches in Recommendation Systems
Neural Network Architecture | Key Features | Domain | Advantages |
---|---|---|---|
Convolutional Neural Networks (CNN) | Process visual data, extract visual features | Image-based recommendations | - Accurate image-based recommendations - Ability to capture visual preferences |
Recurrent Neural Networks (RNN) | Process sequential data, capture temporal dependencies | User behavior, text-based recommendations | - Personalized recommendations based on historical interactions - Ability to understand user preferences over time |
Conclusion
The integration of artificial intelligence (AI) in recommendation systems has revolutionized the digital landscape, bringing personalized and targeted solutions to users. By leveraging machine learning algorithms, collaborative filtering, content-based filtering, and hybrid recommender systems, businesses can enhance customer experiences and drive engagement.
Natural language processing (NLP) techniques further optimize recommendation systems by enabling them to understand and interpret user input. This results in more accurate and contextually relevant suggestions, improving the overall user experience and increasing the likelihood of conversion.
Deep learning advancements have also played a significant role in recommendation systems. Complex neural network architectures, such as Convolutional Neural Networks (CNN) and Recurrent Neural Networks (RNN), capture intricate patterns and relationships in vast amounts of data. This allows for highly personalized recommendations based on individual user preferences, ultimately increasing customer satisfaction.
By embracing AI in recommendation systems, businesses can unlock the potential to deliver personalized experiences, increase customer satisfaction, and drive business growth. With the power of AI, businesses can provide accurate and relevant recommendations that align with user preferences, resulting in a significant impact on sales and user engagement.
FAQ
What is AI in recommendation systems?
AI in recommendation systems refers to the use of artificial intelligence technology to analyze user behavior, preferences, and historical data in order to generate personalized recommendations.
How does AI in recommendation systems work?
AI in recommendation systems utilizes machine learning algorithms, collaborative filtering, content-based filtering, and hybrid recommender systems to analyze data and provide accurate and relevant suggestions to users.
What is the role of NLP in recommendation systems?
Natural language processing (NLP) techniques enable recommendation systems to understand and interpret user input, such as search queries or product descriptions, to generate personalized suggestions.
How does deep learning advancements impact recommendation systems?
Deep learning advancements in recommendation systems enable the development of complex neural network architectures that can capture intricate patterns and relationships in vast amounts of data, resulting in more accurate and personalized recommendations.
Source Links
- https://bigzoon.com/2023/11/25/chat-openai-com-login/
- https://thetradable.com/ai/unlocking-success-how-to-leverage-ai-in-marketing
- https://medium.com/@bhadashot/elon-musk-is-launching-6g-phone-631a5f715dc6
If you want to know other articles similar to Unlocking Potential with AI in Recommendation Systems you can visit the Blog category.
Leave a Reply
Related Post...