
Unlocking the Power of Machine Learning in Finance

The finance industry is becoming increasingly complex with the evolving regulatory environment, globalization, and technological advancements. The traditional methods of data analysis and decision-making are not sufficient anymore. Financial institutions are now relying on machine learning algorithms and artificial intelligence to gain a competitive edge and optimize their operations.
Machine learning in finance involves the use of algorithms to analyze large volumes of data and identify patterns, trends, and anomalies. It can help financial institutions to make informed decisions, enhance risk management strategies, and provide personalized customer experiences.
Artificial intelligence in finance is also transforming the industry. It enables machines to learn from past data and predict future outcomes. It can help financial institutions to automate routine tasks, improve fraud detection, and enhance compliance.
See Also...
Financial machine learning is a subset of machine learning that is specific to the finance industry. It involves applying machine learning algorithms to financial data, such as stock prices, financial statements, and economic indicators, to predict financial outcomes.
Key Takeaways:
- Machine learning in finance is transforming the industry by improving decision-making and boosting efficiency.
- Artificial intelligence in finance enables machines to learn from past data and predict future outcomes.
- Financial machine learning involves applying machine learning algorithms to financial data to predict financial outcomes.
- Financial institutions are relying on machine learning to gain a competitive edge and optimize their operations.
- Machine learning can help financial institutions to automate routine tasks, improve fraud detection, and enhance compliance.
- What is machine learning in finance?
- How is artificial intelligence reshaping the finance industry?
- What is predictive analytics in finance?
- What is algorithmic trading?
- How does financial data science and big data impact finance?
- What is the role of fintech in finance?
- How is machine learning revolutionizing finance?
Reshaping the Finance Industry with Predictive Analytics and Algorithmic Trading
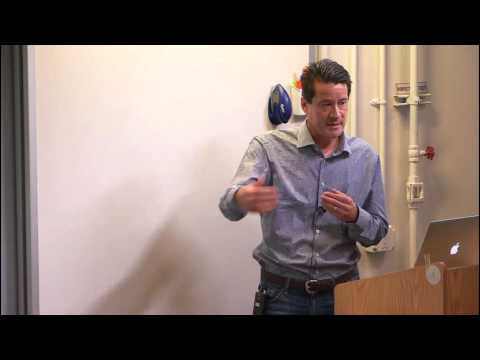
Machine learning and predictive analytics are rapidly transforming the finance industry, with businesses harnessing these technologies to revolutionize the way they operate. One of the most prominent areas where these new technologies are having a substantial impact is in predictive analytics in finance, which is helping financial institutions make informed investment decisions.
Predictive analytics models use data mining, machine learning, and artificial intelligence to analyze vast amounts of data and identify patterns that can aid in forecasting market trends. This process enables financial analysts to make data-driven decisions about investments, potentially leading to higher returns.
See Also...
Additionally, the use of machine learning algorithms is improving the efficiency of trading processes through algorithmic trading. This technology uses advanced algorithms to automate trading processes, enabling institutions to respond quickly to market changes and make more informed trading decisions. The use of algorithmic trading is rapidly increasing, with estimates suggesting that it now accounts for over 70% of all trading in the US.
Advantages of Predictive Analytics and Algorithmic Trading
"Both predictive analytics and algorithmic trading, as applications of machine learning, have been incredibly valuable in the finance industry. They enable businesses to make more informed decisions and automate time-consuming processes, ultimately boosting efficiency and profitability."
One advantage of predictive analytics is its ability to identify patterns and trends that traditional analysis may overlook. Predictive analytics models can factor in a vast array of variables, including market trends, macroeconomic factors, and consumer behavior, to create a more complete picture of the market and identify potential investment opportunities. Additionally, these models can continually learn and adapt to new data, allowing them to become more accurate over time.
See Also...
Algorithmic trading, on the other hand, allows financial institutions to execute trades at a much faster pace than human traders. By utilizing machine learning algorithms, algorithmic trading can quickly analyze market conditions and execute trades at optimal times and prices. This technology enables financial institutions to respond quickly to market changes and make more informed trading decisions.
Challenges in Predictive Analytics and Algorithmic Trading
Despite the many advantages of predictive analytics and algorithmic trading, there are still challenges to their widespread adoption. One of the main challenges is the need for large amounts of high-quality data. Without this data, predictive analytics models may not be able to identify patterns accurately, leading to inaccurate predictions.
Another challenge is the need for skilled professionals to implement and maintain these technologies. Data scientists and financial analysts require a deep understanding of machine learning algorithms to develop accurate models, and financial institutions must invest in training and development programs to retain these skilled professionals.
See Also...
Leveraging Financial Data Science and Big Data in Finance
Financial institutions have historically generated vast amounts of data, including transactional, operational, and customer data. With the advent of big data and the development of advanced machine learning algorithms, financial data science has become critical in unlocking valuable insights and identifying emerging trends in the finance industry. Financial data science refers to the use of data science techniques to uncover insights and make predictions in financial services.
Big data technology is a crucial component of financial data science. Big data refers to the vast amounts of structured and unstructured data generated by financial institutions. Machine learning algorithms can analyze big data, generating insights for financial institutions. Big data can come from a variety of sources, including social media, transactional data, and customer interactions.
Applications of Financial Data Science and Big Data in Finance
Financial data science and big data are used in finance to analyze customer behavior, identify market trends, and develop predictive models. For example, big data can be used to improve fraud detection and prevention strategies. Advanced machine learning algorithms can analyze large amounts of data to identify patterns and anomalies, flagging potential fraud before it occurs.
Big data is also used in credit scoring. Machine learning algorithms can analyze customer data to generate a credit score that can help banks make better lending decisions. Big data is also used to develop predictive models for investment decisions. For example, analytical models can analyze stock market data to identify undervalued stocks, helping traders make more informed investment decisions.
The Role of Fintech in Financial Data Science
Fintech, or financial technology, has made significant contributions to the field of financial data science. Fintech companies specialize in developing software and technology to improve financial services, including data analysis and machine learning algorithms. Fintech has enabled financial institutions to process and analyze vast amounts of financial data quickly and efficiently.
Conclusion
Throughout this article, the transformative role of machine learning in the finance industry has been explored. The use of machine learning algorithms and techniques has reshaped the way financial institutions operate by improving decision-making and increasing efficiency.
The impact of predictive analytics and algorithmic trading was also examined, highlighting the ability to forecast market trends and automate trading processes. The use of financial data science and big data in finance was emphasized, showing how vast amounts of financial data can be analyzed to uncover insights and patterns.
Overall, it is clear that the growing importance of financial technology, quantitative finance, and machine learning algorithms is revolutionizing the finance industry. As advancements in technology continue to progress, the application of machine learning in finance will become increasingly vital.
FAQ
What is machine learning in finance?
Machine learning in finance refers to the use of algorithms and statistical models to enable computers to learn and make predictions or decisions without being explicitly programmed. In the finance industry, machine learning can be applied to various tasks such as risk assessment, fraud detection, and portfolio management.
How is artificial intelligence reshaping the finance industry?
Artificial intelligence, including machine learning, is reshaping the finance industry by enabling financial institutions to automate processes, improve decision-making, and enhance efficiency. AI algorithms can analyze vast amounts of data to uncover valuable insights, predict market trends, and develop more accurate investment strategies.
What is predictive analytics in finance?
Predictive analytics in finance involves using historical and real-time data to generate forecasts and make informed predictions about future market trends, customer behavior, and financial outcomes. By leveraging machine learning algorithms, predictive analytics helps financial professionals identify potential risks and opportunities, guiding more informed decision-making.
What is algorithmic trading?
Algorithmic trading is a method of executing trades in financial markets using pre-programmed instructions run by computer algorithms. These algorithms leverage machine learning to analyze market data, identify patterns, and make trading decisions in real-time. Algorithmic trading aims to enhance trading efficiency, reduce human error, and exploit market inefficiencies.
How does financial data science and big data impact finance?
Financial data science involves the use of techniques from data science in the context of finance. It leverages machine learning algorithms to extract insights, predict future financial trends, and improve decision-making. Big data in finance refers to the vast amount of structured and unstructured data available for analysis. By using big data and financial data science techniques, financial institutions are able to uncover patterns, detect fraud, and gain a deeper understanding of customer behavior.
What is the role of fintech in finance?
Fintech, short for financial technology, refers to the use of innovative technology solutions to improve financial services. In finance, fintech has played a significant role in enabling the processing and analysis of big data, facilitating online payments, developing mobile banking applications, and enhancing the overall user experience. Fintech leverages machine learning and other advanced technologies to transform traditional financial processes.
How is machine learning revolutionizing finance?
Machine learning is revolutionizing finance by improving decision-making, automating processes, and enhancing efficiency. With the ability to analyze large volumes of data quickly, machine learning algorithms can uncover patterns, make predictions, and optimize investment strategies. By leveraging machine learning, financial institutions can gain a competitive edge in a rapidly evolving industry.
If you want to know other articles similar to Unlocking the Power of Machine Learning in Finance you can visit the Blog category.
Leave a Reply
Related Post...